Let’s dive into a topic that’s been buzzing around our circles lately – how to do an A/B test. You know, that seemingly straightforward concept of A/B testing? Or as some like to call it, “split testing.” It’s become the bread and butter for businesses looking to optimize their content. But here’s where I’m going to drop a bombshell: A/B testing, in all its glory, isn’t just about the numbers. In fact, I’d argue that it’s been grossly oversimplified by many.
Now, before you pull up your latest Google Analytics report to prove me wrong, hear me out. I’m not saying that testing isn’t essential. Of course, tests are crucial. They provide businesses with invaluable insights into customer behavior. But there’s a significant piece of the puzzle that’s often overlooked: the human element behind the data.
Many businesses run experiments, eagerly awaiting the results, hoping to see a clear winner. But what if I told you that these tests, while valuable, only scratch the surface? That by merely relying on these numbers, we’re missing out on a deeper, richer understanding of our customers?
It’s a controversial stance, I know. Especially when the digital world is so enamored with data. But I genuinely believe that there’s more to split testing than meets the eye. And that’s what I’m here to unpack with you.
But first, let’s understand what A/B testing actually is.
Table of contents
- Demystifying A/B Testing: The Basics
- A/B Testing vs. Multivariate Testing: What’s the Difference?
- How to do a Split Test: A Deep Dive into the Process
- Analyzing the Data: Frequentist vs. Bayesian Approach
- The Controversial A/B Testing Crossroads: Beyond Traditional Analysis
- Conclusion: Bridging the Gap Between Heart and Numbers
- Bonus: The Eternal Dance: Marrying Qualitative Insights with Quantitative Data in a Continuous A/B Testing Loop
- Frequently Asked Questions
Demystifying A/B Testing: The Basics
A/B testing is right up there near the top of the latest trends in digital marketing and for a good reason. But let’s break it down for a moment. At its core, an A/B test is a method where users are shown two versions of content to determine which one performs better in achieving a specific business goal. Think of it like this: you have two buttons on your website, each with a different design. Which one gets more clicks? That’s where A/B testing comes into play.
You start with your original version, often called the “control.” Then, you introduce a new version with a change – maybe it’s the color of those buttons or a different headline. This is where testing tools become invaluable. They allow businesses to run these experiments, comparing the two approaches to see which resonates more with their target audience. The ultimate aim? Conversion rate optimization. It’s all about improving those rates, whether it’s sales, sign-ups, or any other success metric.
But here’s the thing: A/B testing isn’t just a one-and-done deal. It’s an ongoing process. Once you’ve gathered data from your initial test, you can use those insights to inform future tests. Got a testing idea that didn’t quite hit the mark? No worries. Refine it, test again, and inch closer to that business goal.
A/B Testing vs. Multivariate Testing: What’s the Difference?
Now, let’s dive a bit deeper. While A/B testing is the darling of the digital marketing world, multivariate testing is another player in the game. And trust me, it’s not just a fancy term to throw around at marketing meetings.
So, what’s the deal with multivariate testing? While A/B tests focus on testing two versions of a single element, multivariate tests take it up a notch. They allow you to test multiple key elements simultaneously. Imagine tweaking your headline, button color, and image all at once. That’s multivariate testing in action.
But here’s the catch: while it sounds like a dream come true for any digital marketer, multivariate tests require a much larger sample size to yield actionable insights. You’re juggling multiple variables, after all. And while A/B testing can give you a clear direction on which version works best, multivariate testing can offer a more nuanced understanding of how different elements interact.
However, regardless of whether you’re diving into A/B or multivariate testing, it’s crucial to have clear experiment objectives. Know what you’re aiming for, whether it’s boosting sales, increasing newsletter sign-ups, or any other goal. And remember, always choose the right testing platform for your needs. One that aligns with your experiment variant and overall strategy.
How to do a Split Test: A Deep Dive into the Process
Alright, now that we’ve got a solid grasp on what A/B testing is, let’s roll up our sleeves and dive into the nitty-gritty: how to actually set up an A/B test. Trust me. It’s not as daunting as it might seem, especially if you’re armed with the right knowledge.
First things first, you’ve got to decide on the content you want to test. This could be anything from a landing page headline to the color of a call-to-action button to the calls to action themselves. Those are your starting points. But here’s the kicker: you don’t want to change everything all at once. The beauty of A/B testing lies in its simplicity. You’re comparing the original version of your content with a new version that has one key change. This ensures that any difference in user actions can be attributed to that specific change.
Once you decide on the testing elements, you must create a hypothesis. A hypothesis is a guiding principle for your test, ensuring it has a defined purpose. It sets a clear direction by establishing a specific expected outcome against which the results can be measured.
A hypothesis is fundamental for testing because it establishes the foundation and rationale for the entire experiment. It serves as a predictive statement, guiding researchers in what they anticipate will occur.
Without a hypothesis:
- Lack of Direction: Testing would be aimless. You wouldn’t have a clear understanding of what you’re trying to prove or disprove, making the results ambiguous and potentially irrelevant.
- No Basis for Analysis: Once the test concludes, you’d have no benchmark against which to measure the results. A hypothesis provides that benchmark, allowing for a structured analysis of outcomes.
- Wasted Resources: Running tests without a clear hypothesis can lead to wasted time, effort, and resources. You might end up testing variables that have no significant impact or relevance to your objectives.
- Inability to Draw Conclusions: The end goal of any test is to draw meaningful conclusions that can guide future actions or decisions. Without a hypothesis, it becomes challenging to derive actionable insights from the test results.
- Compromised Credibility: In the scientific and business communities, tests conducted without a clear hypothesis are often viewed with skepticism. They lack the rigor and structure that lend credibility to experimental results.
In essence, you should never run a test without a hypothesis because it’s akin to embarking on a journey without a map. While you might stumble upon interesting findings, you’ll lack the clarity and direction to make the most of your discoveries.
An example hypothesis for your test might be “If we change the call-to-action button color from blue to green on our product page, then we will see a 10% increase in click-through rates over the next 30 days.”
With your hypothesis written, it’s time to choose a testing tool. There are a plethora of tools out there, each with its own unique features. The key is to pick one that aligns with your experiment objectives and caters to your target audience. This tool will allow you to show the current version to half of your real users and the new version to the other half.
Now, let’s talk about the testing journey. It’s not just about setting up the test and waiting for results. It’s about continuously monitoring user interactions, gathering valuable insights, and refining your approach based on deep insights. Remember, A/B testing is as much an art as it is a science.
How to Do an A/B Test and Analyze the Data
- Define Your Testing Elements
Decide on the specific content or feature you want to test and create a hypothesis.
- Choose Your Testing Tool
Pick a tool that aligns with your experiment objectives and caters to your target audience.
- Set Up Your Original Version
This is your control, the current version of your content
- Introduce the Experiment Variant
Make one key, fundamental change to your original version.
- Monitor User Actions
Use your testing tool to track how users interact with both versions.
- Gather and Analyze Data
Dive deep into the results to gain valuable insights into user behavior.
Analyzing the Data: Frequentist vs. Bayesian Approach
Now, when it comes to data analysis, there are two primary schools of thought: the frequentist approach and the Bayesian approach. And trust me, understanding the difference between the two can make or break your testing process.
The frequentist approach is all about long-term frequency. It looks at the likelihood of an event happening based on repeated trials. So, if you’re using the frequentist approach in A/B testing, you’re essentially asking: “If I ran this test over and over again, what’s the probability that version A would outperform version B?” The frequentist approach is all about probabilities and long-term predictions.
On the other hand, the Bayesian approach is a bit more dynamic. It combines prior knowledge with current observed data. So, in the context of A/B testing, the Bayesian approach would consider any prior tests or data you have and combine it with the results of your current test. The Bayesian approach is adaptive, updating its predictions as more data becomes available.
Both approaches have their merits. The frequentist approach is straightforward and based on hard probabilities, while the Bayesian approach offers a more adaptive and holistic view. The key is to understand the nuances of each and choose the one that aligns best with your testing objectives and the nature of your data.
The Controversial A/B Testing Crossroads: Beyond Traditional Analysis
Alright, friend, here’s where we venture into the deep waters. After diving into the intricacies of data analysis, from the frequentist to the Bayesian approach, I’ve got a revelation that might ruffle some feathers. Now, I know we’ve been singing praises of data and its undeniable power in shaping our decisions. But here’s my controversial take: data isn’t the be-all and end-all. In fact, I’d argue that in our obsession with numbers, we’re missing out on a crucial piece of the puzzle.
Now, before you raise your eyebrows and question my sanity, let me clarify. I’m not dismissing the importance of data. Far from it. But what if, in our relentless pursuit of quantitative insights, we’re overlooking the qualitative? What if the real magic lies not just in the numbers but in the stories they tell, the emotions they evoke, and the human experiences they represent?
I can almost hear the gasps from the data purists. But stick with me. As we delve deeper into this controversial terrain, I promise to shed light on a perspective that challenges the status quo and invites you to think differently about the world of A/B testing.
The Facts
- The Emotional Connection: Let’s take a trip down memory lane. Remember that ad we couldn’t stop talking about? The one that gave us goosebumps, made us laugh, or even shed a tear? It wasn’t just the visuals or the catchy jingle; it was the emotion it evoked. Similarly, in the realm of A/B testing, it’s not just about which button color or headline variation gets more clicks. It’s about understanding the deeper emotional triggers that drive those decisions. Why does one version resonate more than the other? It’s often tied to the emotions and experiences of the users. By tapping into these emotions, businesses can create content that not only drives conversions but also fosters genuine connections.
- Beyond Surface-Level Metrics: In our data-driven world, it’s easy to get caught up in the numbers. Click-through rates, bounce rates, conversion rates – these metrics are crucial, no doubt. But they’re just the beginning. What about the long-term engagement, the repeat visits, the word-of-mouth referrals? What about brand loyalty or customer lifetime value? These are metrics that often get overshadowed in the immediate rush for conversions. But they provide a richer, more holistic understanding of user behavior. By looking beyond the immediate metrics, businesses can craft strategies that foster long-term relationships and sustained growth.
- The Power of Anecdotal Evidence: Here’s a story for you. I once ran an A/B test for a client. Version A had a slightly higher conversion rate, but Version B had an overwhelming amount of positive feedback in the comments section. Now, if we were to go by numbers alone, Version A would be the clear winner. But the qualitative feedback from Version B provided invaluable insights into user preferences and pain points. This is the power of anecdotal evidence. While numbers provide a quantitative measure, stories and feedback capture the qualitative nuances that numbers alone can’t. They offer a window into the user’s world, their challenges, desires, and motivations. By valuing and integrating this feedback, businesses can create content that truly resonates with their audience.
In the vast landscape of A/B testing, it’s easy to get lost in the numbers. But by blending the art of understanding people with the science of data, businesses can craft strategies that are not only effective but also deeply human-centric. After all, at the heart of every click, every conversion is a human being with emotions, desires, and stories. And it’s these stories that make all the difference.
Opposing Arguments: The Other Side of the A/B Testing Coin
- Data is King: In the corridors of modern business, there’s a mantra that’s often chanted: “Data is King.” And there’s a solid reason for this belief. Many argue that in our digital age, where every click, every interaction can be tracked and analyzed, numbers offer the most objective measure of success. They contend that emotions and anecdotes are subjective, prone to bias, and can’t be generalized. Data, on the other hand, doesn’t lie. It provides a clear, unbiased view of user behavior, allowing businesses to make informed decisions. In a world where margins are thin and competition is fierce, can businesses really afford to rely on anything other than hard, quantifiable data
- Anecdotal Evidence is Unreliable: Here’s another contention that’s hard to ignore. Critics often point out that while stories and anecdotes are compelling, they’re inherently unreliable. After all, they’re based on individual experiences, which can be influenced by a myriad of factors. Can a handful of user feedback really be compared to the insights gleaned from thousands, if not millions, of data points? Opponents of the anecdotal approach argue that while these stories offer depth, they lack the breadth and scale that data provides. For them, making business decisions based on a few anecdotes is akin to navigating treacherous waters without a compass.
While these opposing arguments offer valid points, it’s essential to remember that in the world of A/B testing, it’s not about choosing one over the other. It’s about finding a balance. Data provides the breadth, the scale, and the objectivity, while anecdotes offer depth, nuance, and context. By integrating both, businesses can craft strategies that are not only effective but also deeply resonant.
Conclusion: Bridging the Gap Between Heart and Numbers
As we journey through the intricate maze of A/B testing, one thing becomes abundantly clear: it’s not a battle between data and stories, but a dance. A harmonious ballet where numbers set the rhythm and emotions paint the narrative.
Imagine, for a moment, a world where businesses solely rely on data. Decisions are made, strategies are crafted, all based on cold, hard numbers. But in this world, something is amiss. The human touch, the emotional connection, the very essence that makes brands resonate with their audience, is lost.
Now, envision a different scenario. A world where businesses are swayed solely by stories, by the emotional feedback of a few. While this world is rich in depth and nuance, it lacks the scale, the objectivity that data provides. Decisions are made based on feelings, which, while powerful, can sometimes lead astray.
So, how do we bridge this divide? How do we marry the quantitative breadth of data with the qualitative depth of stories?
The answer lies in integration. Start with the data. Let it guide you, provide you with a broad overview of user behavior. But don’t stop there. Dive deeper. Seek out the stories, the feedback, the emotions behind the numbers. Use qualitative insights to add layers, context, and depth to the quantitative data.
And as you weave these two threads together, a clearer picture emerges. One where strategies are not only effective but also deeply resonant. One where businesses don’t just chase conversions but foster genuine connections.
In the end, it’s about striking a balance. A balance where numbers meet narratives, where data dances with emotions. And in this harmonious ballet, businesses don’t just succeed; they thrive, resonate, and truly connect.
Bonus: The Eternal Dance: Marrying Qualitative Insights with Quantitative Data in a Continuous A/B Testing Loop
Picture this: a dance that never ends, a cycle that perpetually refines itself, drawing from the heart’s whispers and the mind’s clarity. This is the dance of A/B testing, where qualitative insights and quantitative data are forever intertwined in a rhythmic loop.
1. Begin with the Heartbeat: Qualitative Insights to Identify Potential Tests
Every dance begins with a beat, a rhythm that sets the pace. In our process, this beat is the qualitative insight. Dive deep into the stories, the feedback, the emotions of your users. What are they saying? What are they feeling? Generally, these narratives, these whispers of the heart, will guide you, pointing towards potential areas of exploration. Maybe it’s a button that doesn’t resonate. Or perhaps it’s a piece of content that evokes strong emotions. Listen to these stories, for they will illuminate the path ahead.
2. The Dance of Exploration: Performing the Test
With potential tests identified, it’s time to step onto the dance floor. This is where quantitative data takes the lead. Craft your A/B test based on the insights gleaned from the stories. Introduce the changes, set up your control, and let the dance begin. Monitor user interactions, track conversions, and gather data. This phase is all about exploration, about seeing how the changes resonate on a broader scale.
3. The Reflection: Merging Heart and Mind
Once the test concludes, it’s time for reflection. Dive into the data, but don’t lose sight of the stories. How did the changes impact user behavior? Did the quantitative results align with the qualitative insights? This phase is a beautiful blend of heart and mind, where numbers meet narratives. It’s about understanding not just the ‘what’ but also the ‘why’.
4. The Renewal: Qualitative Insights for the Next Dance
As the reflection phase concludes, the dance doesn’t end. Instead, it evolves. Return to the stories, to the qualitative insights. What are users saying now? How have their emotions and feedback shifted post-test? These renewed insights will guide you, pointing towards new areas of exploration, new potential tests. And thus, the dance begins anew.
In this eternal dance, there’s no beginning or end, just a continuous loop of exploration, reflection, and renewal. It’s a process that ensures businesses are always in tune with their users, always evolving, always resonating. And in this harmonious ballet, brands don’t just connect; they create magic.
Frequently Asked Questions
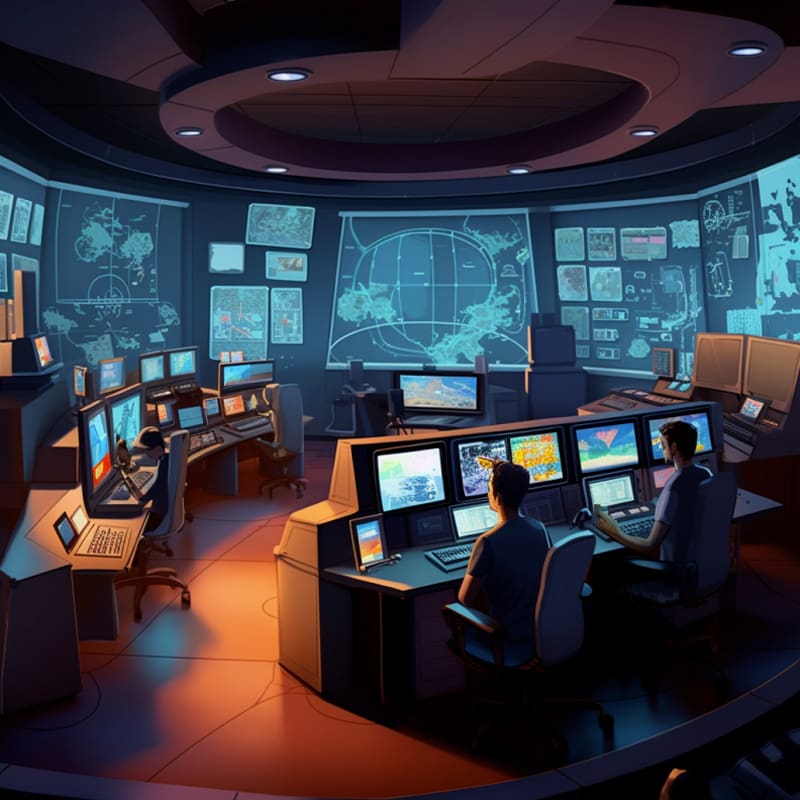
A/B testing, often referred to as split testing, is a method of comparing two versions of a webpage or app against each other to determine which one performs better in terms of a specific objective, be it conversions, click-through rates, or any other metric.
In A/B testing, you take the current version of your content (known as the control) and compare it against a new version with one key change (the variant). Half of your users see the original, and the other half see the new version. By monitoring user interactions, you can determine which version resonates more with your audience.
The human element brings depth, context, and emotion to the table. While data can tell you what is happening, the human element provides insights into why it’s happening. Understanding emotions, feedback, and stories of users ensures that tests are rooted in genuine user needs and desires.
Balancing data-driven decisions with the human element involves integrating quantitative data with qualitative insights. While data provides a broad overview, qualitative insights offer depth and nuance. Together, they ensure a holistic understanding of user behavior.
A hypothesis is an educated guess or prediction about the potential outcome of your test. It sets the direction for your A/B test, outlining what you expect to happen.
The hypothesis provides a clear direction for your test. It ensures that your test has a purpose and that the results can be measured against a specific expected outcome.
Interpreting results involves analyzing the data, understanding the difference in performance between the original and the variant, and integrating qualitative feedback. It’s crucial to ensure statistical significance and consider both the frequentist and Bayesian approaches.
The frequentist approach focuses on long-term frequency, looking at the likelihood of an event happening based on repeated trials.
The Bayesian approach combines prior knowledge with current observed data, updating predictions as more data becomes available.
Common mistakes include not waiting for statistical significance, testing too many elements at once, and not considering external factors that might influence results.
You can test various elements online, including headlines, call-to-action buttons, images, content layout, and more. The key is to ensure that each test focuses on one specific change to accurately measure its impact.
Last Updated on August 14, 2023 by Benjamin Teal